Does deforestation increase malaria rates? Not in Africa, surprisingly
This blog updates a blog previously published on the Center for Global Development website.
Deforestation isn’t associated with higher malaria prevalence in children in 17 African countries. Nor is it associated with higher fever in children in 41 countries across Africa, Asia, and Latin America. That’s the surprising conclusion of our recently published paper in World Development.
This means that, at least in Africa where 88% of malaria cases occur, public health efforts to reduce malaria should continue to focus on proven anti-malarial interventions. These include insecticide-treated bed nets, indoor spraying, housing improvements, and prompt clinical treatment, which along with other interventions have reduced the incidence of this killer disease by 41% between 2000-2015.
For advocates of forest conservation in Africa, there are many good reasons to keep forests standing. These include carbon storage, biodiversity habitat, and clean water provision, alongside other goods and services. However, forest conservation might not have anti-malarial benefits, at least not in Africa.
A surprising finding
These results, which we first released last year in a Center for Global Development working paper, may come as a surprise to people following the literature on deforestation and malaria. Certainly, it is well established that deforestation can increase malaria risk factors in some settings. Relative to forests, deforested lands have been found to have higher temperatures, more sunlight, and more standing water, which favor some types of malaria-transmitting mosquitoes. And relative to forests, cleared lands have also been found to have fewer insectivores, more species competing for ecological niche, and arguably fewer “dead-end hosts” to dilute malaria. Furthermore, “frontier malaria” can result from the unstable socio-economic conditions associated with deforestation in many parts of the world, including rapid in-migration, new human exposure and low immunity, poor housing quality, and sparse availability of health services.
But increased malaria risk might not necessarily translate to higher rates of malaria in humans (i.e., “prevalence”). That’s because there’s considerably nuance in the effects listed above. For example, deforested areas may be favored by some mosquito species but not others; deforestation is generally considered to increase the density of malaria-transmitting mosquitoes in Africa and Latin America but decrease their density in Asia. In addition, many other factors besides deforestation also affect malaria prevalence in humans, including climate, community demographics, access to health facilities, and people’s behaviors to avoid malaria.
Eleven previous peer-reviewed studies have compared deforestation to malaria prevalence in humans (see table below). These studies generally analyzed small amounts of municipal-level data from a handful of countries—seven from the Brazilian Amazon, and one each from Indonesia, Malaysia, and Paraguay, as well as one study that compared national-level statistics across 67 countries. Most, though not all, found that more deforestation is associated with more malaria. So, it was a surprise to find no association between deforestation and malaria in our study.
So, why might studies find that deforestation leads to higher malaria rates in South America and Southeast Asia but not in Africa? The explanation, we speculate in our paper, may have something to do with differences between how deforestation happens in Africa versus elsewhere. Deforestation in Africa is largely driven by the steady expansion of rotational agriculture for domestic use by long-time smallholder farmers in stable socio-economic settings where malaria is already endemic and previous exposure is high. In contract, deforestation in much of Latin America and Asia is driven by rapid clearing for market-driven agricultural exports by new frontier migrants without previous exposure. We hope that these hypotheses can be supported or refuted by future work.
How we got there
We came to our conclusions by assembling massive data sets on deforestation and malaria. Our data set on deforestation included annual tree-cover loss between 2001-2015 in 1.5 million ~5.5-kilometer grid-cells across the tropics, compiled from Global Forest Watch. We also obtained data from malaria tests of around 60,000 children in rural Africa and fever recall surveys of around 470,000 children across the rural Tropics conducted under the auspices of USAID’s Demographic and Health Surveys. We combined these two data sets in a multivariate regression analysis that also considered temperature, precipitation, housing quality, water source, access to health services, child age, and bed-net usage.
In addition to our main comparison of deforestation and malaria, we also tested hypotheses generated in advance from previous studies. Did smaller cuts lead to more malaria on a per-hectare basis than larger cuts? No. Did deforestation have a bigger effect in places with more forest? No. Did deforestation have a bigger effect on fever in African and Latin America than Asia? No.
We’d originally also planned to compare the cost-effectiveness of preventing malaria through forest conservation to the cost-effectiveness of common interventions such as bed nets and spraying, as measured in disability-adjusted life years (DALY) per dollar. But since deforestation wasn’t found to affect malaria rates, the DALY-per-dollar benefit was essentially zero.
Bolstering credibility with a pre-analysis plan
We expected our findings were bound to be controversial, no matter what we found. A previous study of deforestation and malaria in the Brazilian Amazon generated some heated back-and-forth. So to bolster the integrity and credibility of our research we used a pre-analysis plan. That is, we wrote down and time-stamped all our hypotheses, methods, models, and variables in advance. Then we stuck with them.
Pre-analysis plans are common and even required for some types of clinical research. But they are still new to social sciences, including economics, where common research practice often involves testing many possible combinations of variables and model specifications. If the authors of such a study only report tests showing favorable results while relegating the results of other tests to the digital trash bin (“data mining” or “p-hacking”), they can inadvertently or deliberately place a thumb on the scale to achieve desired results. This is what we wanted to avoid by writing and following a pre-analysis plan. We published our pre-analysis plan on the Registry for International Development Impact Evaluations (RIDIE) website (available in two parts, here and here).
We offer seven reflections on using a pre-analysis plan in a companion blog, here.
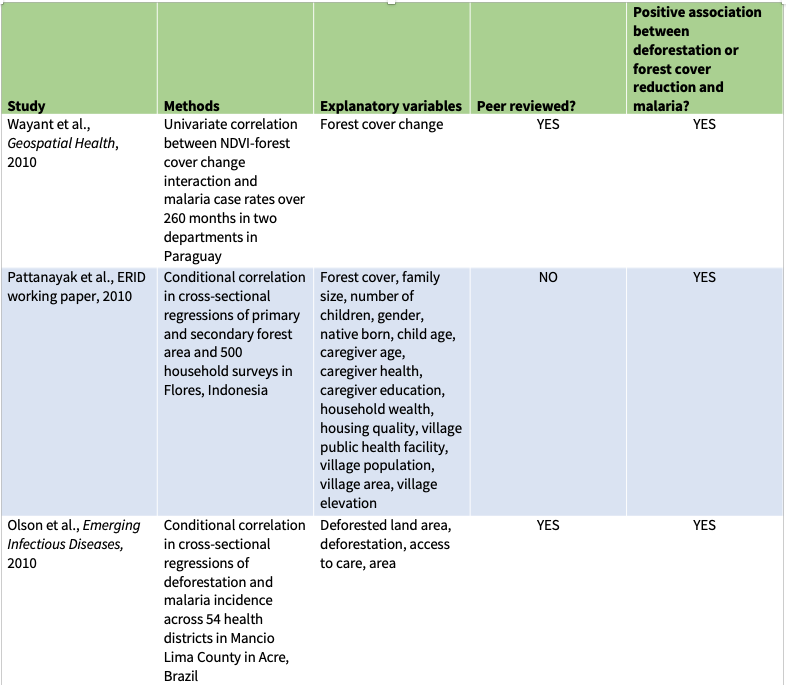
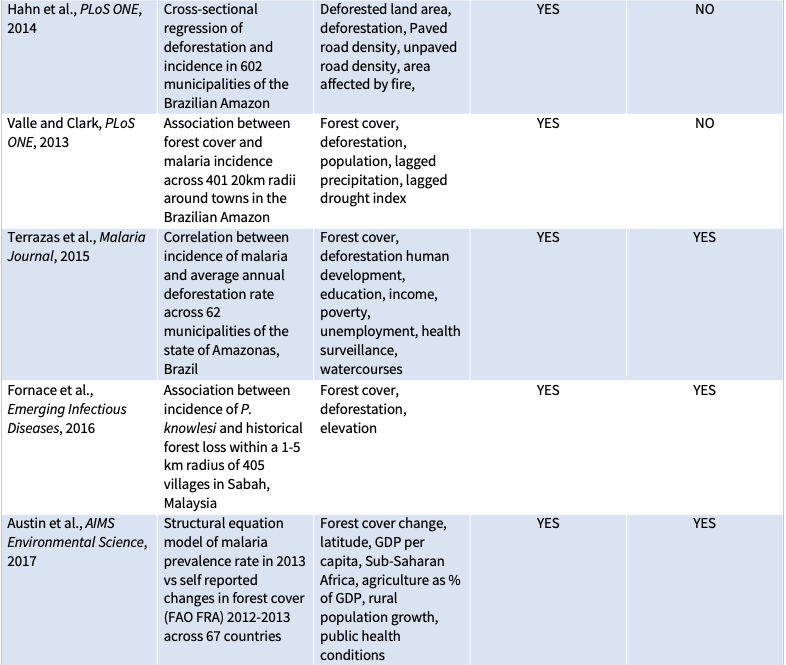
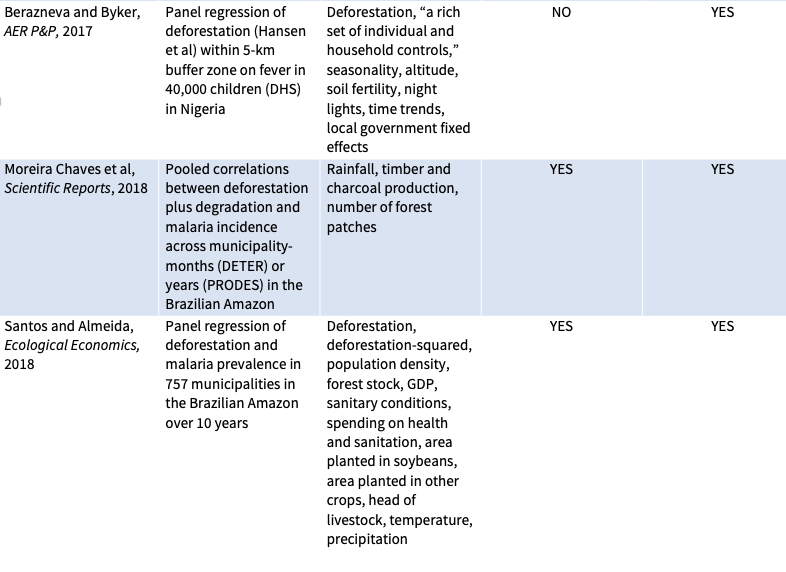
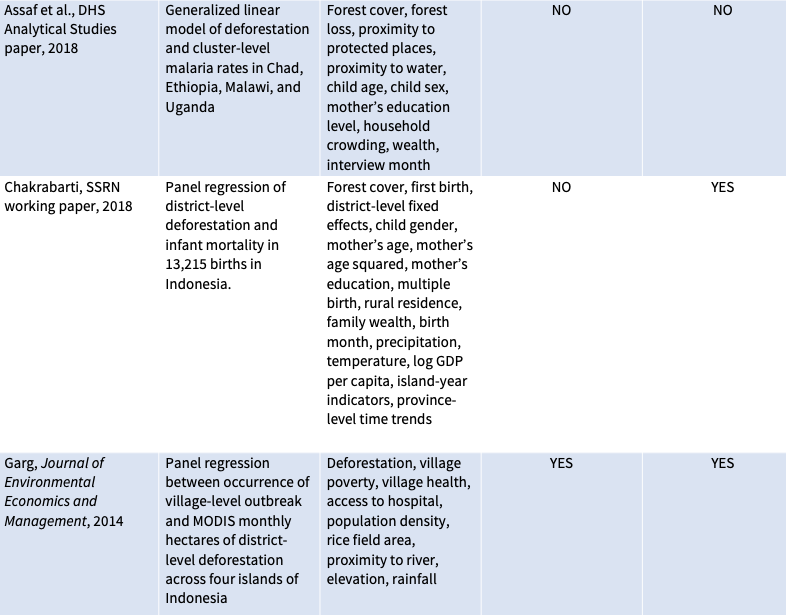
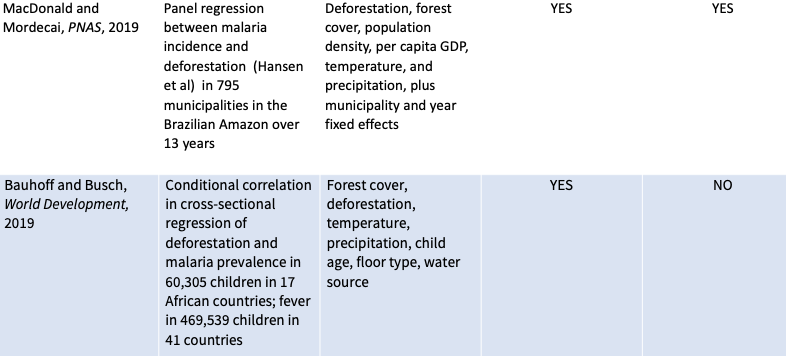